Machine Deep Learning
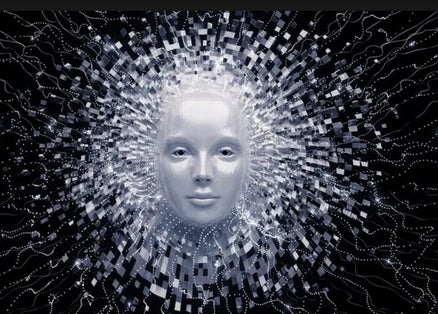
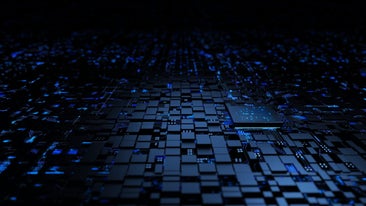
Machine Deep Learning
Machine Deep Learning is the most advanced type of artificial intelligence machine learning technique. It uses computer algorithms to enable computers to learn by example much like people. By using deep learning, a computer model learns to perform classification tasks directly from images, text, or sound. Deep learning models can achieve state-of-the-art accuracy, sometimes exceeding human-level performance. Models are trained by using a large set of labeled data and neural network architectures that contain many layers.
Deep Learning is a powerful and dynamic technology that is allowing the machines to make autonomous decisions. Deep Learning is modelled after the human brain that is able to take decisions that are autonomous in nature. The nervous system of the human body comprises of many neurons that are able to learn the information that is provided to them through various stimuli and, therefore, take necessary steps. For example, if you accidentally touch something hot for the first time as a child, your neurons are trained to understand that touching something hot will be painful. Therefore, you are trained subconsciously to keep your hands off the gas stove.
As a matter of fact, the structure of a neural network is modelled after the neurons in the human brain. It comprises many layers of neurons that are structured to transmit information from the input layer to the output layer. Between the input and the output layer, there are hidden layers present. These hidden layers can be many or just one. A simple neural network comprising of a single hidden layer is known as Perceptron.
In the above diagram for a simple neural network, there is an input layer that takes the input in the form of a vector. Then, this input is passed to the hidden layer which comprises of various mathematical functions that perform computation on the given input. For example, given the images of cats and dogs, our hidden layers perform various mathematical operations to find the maximum probability of the class our input image falls in. This is an example of binary classification where the class, that is, dog or cat, is assigned its appropriate place.
There are two main important operations that are performed in deep learning — classification and regression. The above example is of classification operation. Whereas, regression is the forecast of future events. For example, forecasting the stock prices based on the historical listings as an example of regression.
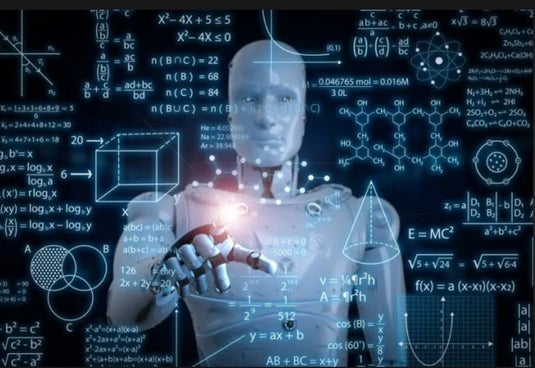
Machine Learning
Machine Learning is concerned with giving machines the ability to learn by training algorithms on a huge amount of data. It makes use of algorithms and statistical models to perform a task without needing explicit instructions.
There are three types of learning here:
- Supervised and semi-supervised learning
- Unsupervised learning
- Reinforcement learning
Machine Learning often deals with the following issues:
- Collecting data
- Filtering data
- Analyzing data
- Training algorithms
- Testing algorithms
- Using algorithms for future predictions
Common examples of this phenomenon are virtual personal assistants, refined search engine results, image recognition, and product recommendations.
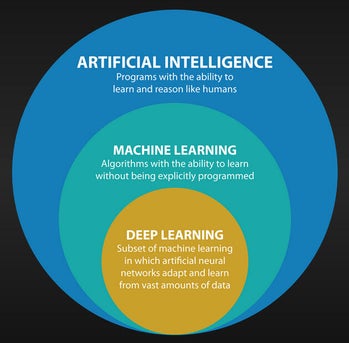
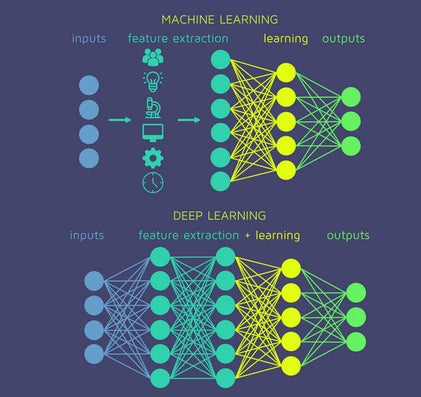
Deep Learning is different from Machine Learning
Deep Learning is an extension of the current Machine Learning algorithms. It has broadened the scope of Machine Learning to incorporate computation and learning of massive datasets. While the traditional machine learning algorithms like K-means clustering, Linear and Multivariate Regression, Logistic Regression and Decision Trees were capable of finding patterns within the data, the accuracy was not always high. However, with the introduction of Deep Learning, things have changed drastically.
Deep Learning incorporates various procedures and methodologies for training systems to learn data and perform suitable predictions on the basis of patterns that are discovered. Deep Learning uses advanced paradigms that were not previously used in Machine Learning. For example, traditional machine learning algorithms used statistical analysis to find patterns in the data. This could generate insights about the data to a given extent, but not more than that. That is, its performance would increase with increment in data but would stagnate after some time.
Deep Learning algorithms like artificial neural networks are able to take up a large amount of data and process it to produce highly accurate results. These neural networks can be fine-tuned to provide better results. For example, you can increase the number of hidden layers in the neural network to increase its accuracy. This allows the neural networks to capture a large number of intricate patterns that the traditional machine learning algorithm cannot capture. This gives them an edge over conventional machine learning.
Therefore, it is best concluded that deep learning is a much better alternative over machine learning as it facilitates a high accuracy rate and faster computation. In summary, Deep Machine Learning is an advanced extension of Machine Learning. Deep Learning is part of Machine Learning because both share the same goal. However, they employ different methodologies to reach that goal.
Deep learning is a specialized form of machine learning. A machine learning workflow starts with relevant features being manually extracted from images. The features are then used to create a model that categorizes the objects in the image. With a deep learning workflow, relevant features are automatically extracted from images. In addition, deep learning performs “end-to-end learning” – where a network is given raw data and a task to perform, such as classification, and it learns how to do this automatically.
Another key difference is deep learning algorithms scale with data, whereas shallow learning converges. Shallow learning refers to machine learning methods that plateau at a certain level of performance when you add more examples and training data to the network.
A key advantage of deep learning networks is that they often continue to improve as the size of your data increases.
In machine learning, you manually choose features and a classifier to sort images. With deep learning, feature extraction and modeling steps are automatic.
Machine Deep Learning can achieve extraordinary results
Deep learning achieves recognition accuracy at higher levels than ever before. This helps consumer electronics meet user expectations, and it is crucial for safety-critical applications like driverless cars. Recent advances in deep learning have improved to the point where deep learning outperforms humans in some tasks like classifying objects in images.
While deep learning was first theorized in the 1980s, there are two main reasons it has only recently become useful:
- Deep learning requires large amounts of labeled data. For example, driverless car development requires millions of images and thousands of hours of video.
- Deep learning requires substantial computing power. High-performance GPUs have a parallel architecture that is efficient for deep learning. When combined with clusters or cloud computing, this enables development teams to reduce training time for a deep learning network from weeks to hours or less.
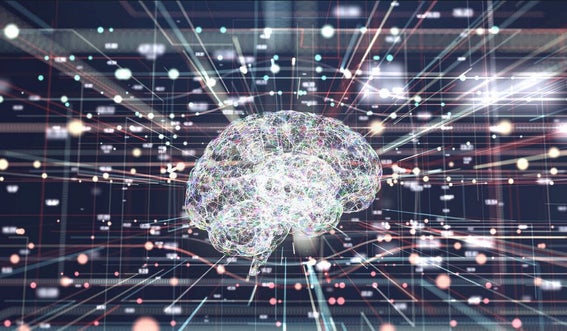
Examples of Deep Learning at Work
Deep learning applications are used in industries from automated driving to medical devices.
Automated Driving: Automotive researchers are using deep learning to automatically detect objects such as stop signs and traffic lights. In addition, deep learning is used to detect pedestrians, which helps decrease accidents.
Aerospace and Defense: Deep learning is used to identify objects from satellites that locate areas of interest, and identify safe or unsafe zones for troops.
Medical Research: Cancer researchers are using deep learning to automatically detect cancer cells. Teams at UCLA built an advanced microscope that yields a high-dimensional data set used to train a deep learning application to accurately identify cancer cells.
Industrial Automation: Deep learning is helping to improve worker safety around heavy machinery by automatically detecting when people or objects are within an unsafe distance of machines.
Electronics: Deep learning is being used in automated hearing and speech translation. For example, home assistance devices that respond to your voice and know your preferences are powered by deep learning applications.
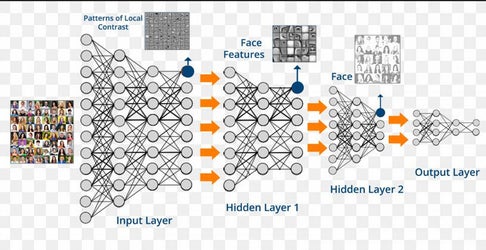
Neural Network Architectures
Most deep learning methods use neural network architectures, which is why deep learning models are often referred to as deep neural networks.
The term “deep” usually refers to the number of hidden layers in the neural network. Traditional neural networks only contain 2-3 hidden layers, while deep networks can have as many as 150.
Deep learning models are trained by using large sets of labeled data and neural network architectures that learn features directly from the data without the need for manual feature extraction.
Convolutional Neural Networks (CNN)
One of the most popular types of deep neural networks is known as convolutional neural networks (CNN or ConvNet). A CNN convolves learned features with input data, and uses 2D convolutional layers, making this architecture well suited to processing 2D data, such as images.
CNNs eliminate the need for manual feature extraction, so you do not need to identify features used to classify images. The CNN works by extracting features directly from images. The relevant features are not pretrained; they are learned while the network trains on a collection of images. This automated feature extraction makes deep learning models highly accurate for computer vision tasks such as object classification.
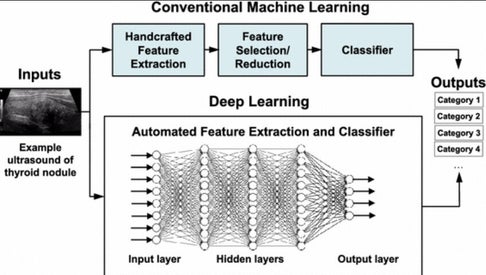
CNNs learn to detect different features of an image using tens or hundreds of hidden layers. Every hidden layer increases the complexity of the learned image features. For example, the first hidden layer could learn how to detect edges, and the last learns how to detect more complex shapes specifically catered to the shape of the object we are trying to recognize.
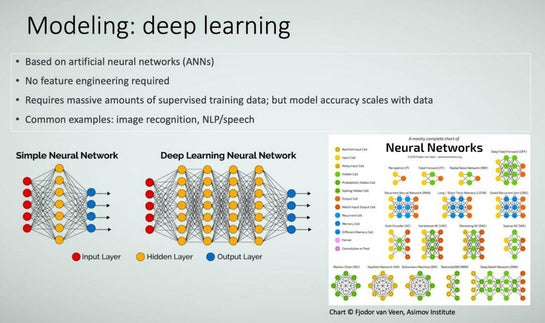
Choosing Between Machine Learning and Deep Learning
Machine learning offers a variety of techniques and models you can choose based on your application, the size of data you're processing, and the type of problem you want to solve. A successful deep learning application requires a very large amount of data (thousands of images) to train the model, as well as GPUs, or graphics processing units, to rapidly process your data.
When choosing between machine learning and deep learning, consider whether you have a high-performance GPU and lots of labeled data. If you don’t have either of those things, it may make more sense to use machine learning instead of deep learning. Deep learning is generally more complex, so you’ll need at least a few thousand images to get reliable results. Having a high-performance GPU means the model will take less time to analyze all those images.
Creating and Training Deep Learning Models: The three most common ways to use deep learning to perform object classification
Training from Scratch
To train a deep network from scratch, you gather a very large labeled data set and design a network architecture that will learn the features and model. This is good for new applications, or applications that will have a large number of output categories. This is a less common approach because with the large amount of data and rate of learning, these networks typically take days or weeks to train.
Transfer Learning
Most deep learning applications use the transfer learning approach, a process that involves fine-tuning a pretrained model. You start with an existing network, such as AlexNet or GoogLeNet, and feed in new data containing previously unknown classes. After making some tweaks to the network, you can now perform a new task, such as categorizing only dogs or cats instead of 1000 different objects. This also has the advantage of needing much less data (processing thousands of images, rather than millions), so computation time drops to minutes or hours.
Transfer learning requires an interface to the internals of the pre-existing network, so it can be surgically modified and enhanced for the new task.
Feature Extraction
A slightly less common, more specialized approach to deep learning is to use the network as a feature extractor. Since all the layers are tasked with learning certain features from images, we can pull these features out of the network at any time during the training process. These features can then be used as input to a machine learning model such as support vector machines (SVM).
Accelerating Deep Learning Models with GPUs
Training a deep learning model can take a long time, from days to weeks. Using GPU acceleration can speed up the process significantly. Using a GPU reduces the time required to train a network and can cut the training time for an image classification problem from days down to hours.
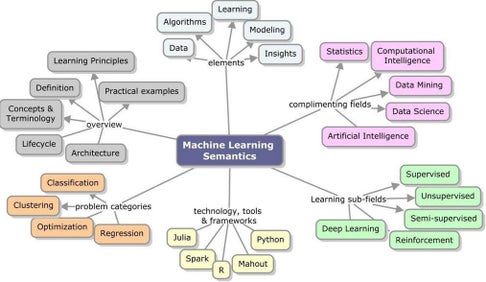
Deep Learning
Being a relatively new field of Machine Learning, Deep Learning is growing faster than ever. Deep Learning is a powerful and dynamic technology that is allowing the machines to make autonomous decisions. There are several instances where you experience Deep Learning in your daily life.
For example, your mobile phone is able to unlock itself after recognizing your face. You are informed about the current weather through conversation with Google Now. Furthermore, you are able to get future insights about the stock market, allowing you to make careful investments. All these are powered by Deep Learning, a revolutionizing technology that has transformed industries around the world.
How Deep Learning works
Generally speaking, Deep Learning is modelled after the human brain that is able to take decisions that are autonomous in nature. The nervous system of the human body comprises of many neurons that are able to learn the information that is provided to them through various stimuli and, therefore, take necessary steps. For example, if you accidentally touch something hot for the first time as a child, your neurons are trained to understand that touching something hot will be painful. Therefore, you are trained subconsciously to keep your hands off the gas stove.
As a matter of fact, the structure of a neural network is modelled after the neurons in the human brain. It comprises many layers of neurons that are structured to transmit information from the input layer to the output layer. Between the input and the output layer, there are hidden layers present. These hidden layers can be many or just one. A simple neural network comprising of a single hidden layer is known as Perceptron.
Neural Network Layers
For a simple neural network, there is an input layer that takes the input in the form of a vector. Then, this input is passed to the hidden layer which comprises of various mathematical functions that perform computation on the given input. For example, given the images of cats and dogs, our hidden layers perform various mathematical operations to find the maximum probability of the class our input image falls in. This is an example of binary classification where the class, that is, dog or cat, is assigned its appropriate place.
There are two main important operations that are performed in deep learning — classification and regression. The above example is of classification operation. Whereas, regression is the forecast of future events. For example, forecasting the stock prices based on the historical listings as an example of regression.
Machine Learning
We can teach a computer to learn by using data, and this process is called machine learning, which uses statistics.
It involves using a computer to solve a problem without being explicitly programmed to do so. The machines can do this by detecting statistical patterns in data.
Essentially, with machine learning, we use existing data and a training algorithm to learn a model of the data. We can then feed new data into that model that it’s never seen before and make predictions about the latest data.
A machine learning model is essentially a function. It’s simply a mapping from an input to an output. In this case, it takes data as an input and produces a prediction as an output.
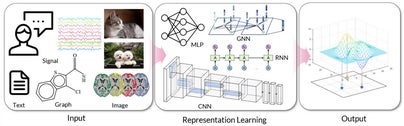
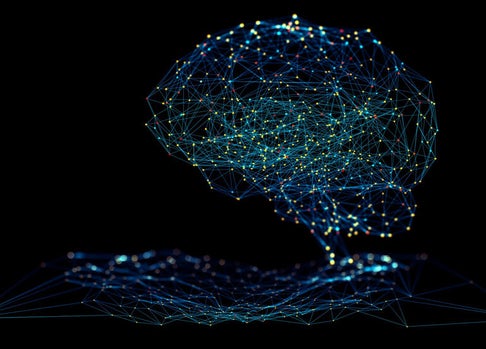
Machine Learning Process
This learning process works because datasets contain the lengths and heights of a selected group of products, which is labeled.
Next, we would feed these data into a machine learning training algorithm. The algorithm would inspect each row of data containing the length, height, and type of product.
The algorithm would learn how length and height correlate with every kind of product. The machine learning training algorithm captures the statistical relationship as a mathematical model.
Finally, if we’ve done everything correctly, we can use this data to make predictions. We can feed this model a new product now seen before, and the model will tell us whether the new product is A or B.
This is a vastly oversimplified explanation of machine learning. However, it captures the essence of what it is that we’re attempting to accomplish.
We feed a set of data into a training algorithm. The algorithm produces a model, then we provide new previously unseen data into that model, and the model will predict what the data represent.
Applying Machine Learning
However, real‑world problems typically involve a lot more columns and rows of data. Besides, they usually include solving much more complicated issues in classifying products.
There are a lot of tasks that we can perform with machine learning, such as classification, regression, clustering, and anomaly detection.
Classification is where we make a decision or prediction involving two or more categories or outcomes, such as deciding whether to accept or reject a specific result based on the input.
Regression is where we attempt to predict a numeric outcome based on one or more input variables, indicating the price of a house based on its features and the sale price of similar homes.
Clustering is where we group objects together based on similarities in their data, which includes grouping customers into marketing segments based on their consumption patterns.
Anomaly detection is where we find observations in the data that are different from the expected data. Detecting a varying number of negative comments about a support case was opened by a customer.
Machine learning is artificial intelligence that gives machines the power to identify patterns, make decisions, and predict outcomes using data alone. It is a powerful tool for helping you and your business make better decisions, create more intelligent products, and automate manual labor.
In addition, traditional machine learning has some severe limitations in the complexity of problems it can solve. To overcome these limitations, we need to learn more advanced tools like deep learning and reinforcement learning.
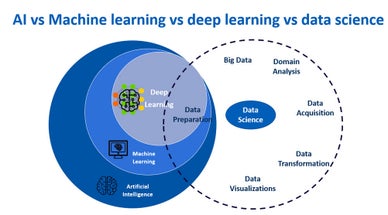
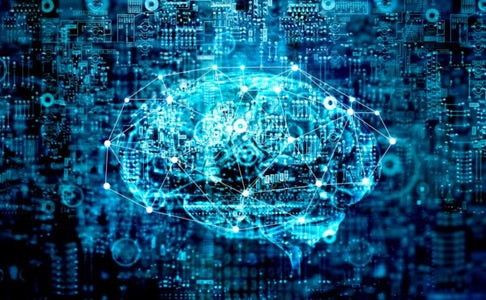
AI Deep Machine Learning Models
Deep learning is a new type of data‑driven AI. It stacks multiple layers of machine learning models, one on top of the other.
This allows it to learn more complex inputs and produce more complex outputs. We typically create deep learning models using a neural network, a graph of nodes and edges based roughly on the organization of neurons in an organic brain.
Deep Learning Process
The nodes represent neurons in the brain. The edges represent the connections between the neurons. First, we feed data into the neural network via its input neurons, on which mathematical operations run on the data in each of the neurons.
Then, each neuron forwards its resulting value to all of the other neurons connected to it. The process is executed multiple times for all nodes in the hidden layer of the network and all the edges in the hidden layer.
Finally, the network produces a prediction from its output neurons. There’s a bit of math involved to make this entire process work; however, we’re going to skip over all the math to keep things simple.
Appliance of Deep Learning
A deep neural network is a network with more than one hidden layer. Adding more hidden layers allows the network to model progressively more complex functions.
As a result, the data becomes more abstract with each additional layer in the network. However, we can also flip these deep neural networks around.
This allows us to use abstract inputs, like describing a person’s facial features, to generate complex outputs like entire art patterns programmatically.
Deep learning takes us beyond using simple tabular data as input and producing simple predictions as output. With deep understanding, we can use complex data as input and have complex data as output which includes text, images, audio, video, and others.
Therefore, it is possible to perform object classification, video segmentation, and motion detection in real-time, but we can also generate synthetic output.
Deep learning can provide a pretty powerful tool for automating tasks that involve complex relationships between complex data. However, it still has significant limitations when it comes to solving multi‑step problems. To solve these types of issues, we need to learn about use learning with reinforcement.
Reinforcement Learning
Reinforcement learning is a variation of machine learning that learns to solve problems by successive experimentation. It starts with an agent interacting with an environment. The agent is trying to achieve a multi‑step goal within the environment.
The environment has a state, which the agent can observe. The agent senses a state of the environment through what it sees and understands via other means. The agent has actions that it can take, which modify the state of the domain.
Finally, the agent receives reward signals as it moves closer to its goal. The agent uses these reward signals to determine which actions were successful and which actions were not.
The agent’s objective is to learn how always to choose the right action given any state of the environment that leads it closer to its goal. In recent years, we’ve combined deep learning with reinforcement learning to create deep reinforcement learning.
Today, the most successful reinforcement learning applications involve video games, computer simulations, and toy problems. However, this technology is beginning to move into the world of business. Shortly, you will likely see these algorithms perform various complex, multi‑step tasks in the real world.
Reinforcement learning is artificial intelligence that can solve complex, multi‑step problems on its own. The machines can learn how to solve these problems without humans’ direct help, which is a compelling technology that will likely shape the future of artificial intelligence for many years to come.
Machine learning is not a product that is available to be purchased off the shelf. It is a collection of methodologies and technologies that fall under the same roof and differ from each other but have the ability and potential to offer great actionable insights into any business and organization.
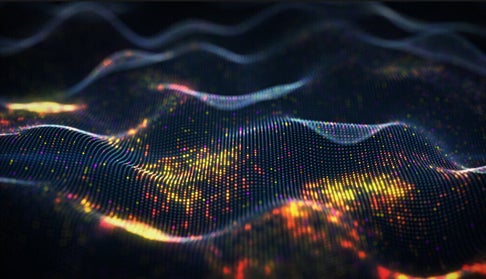
Machine Learning Models
Businesses are incorporating machine learning models to scale and improve by running their operations with software and less human operations — creating controlled scenarios that perform with automatic learning and prediction to make decisions. ML models train data to feed algorithms specific to business activities and variables that change frequently and can impact business effectiveness. Analysts start by identifying the business parts and complex problems that can be solved from data. Then, analyzing the responsiveness of uncontrolled variables to develop and incorporate AI-driven services to its core and scale business operations. Learn more about machine learning models and envision how to extend existing services or develop new solutions.
A Machine learning model is a program that runs an algorithm on a dataset to recognize patterns to learn (train) and reason (logic) from that data to create a clear output (prediction). The ML model training is done incrementally from the data and optimizes the algorithm to find patterns or signals. Framing Machine Learning problems can vary depending on the use case and prediction task. ML models are classified according to how they are trained and data points built to detect patterns. Algorithms are the mathematical procedure used to find patterns in a dataset. Discovering the right algorithms is a key factor in developing ML models. Learn more about the types of algorithms.
How to Build a Machine Learning Model
Training a Machine Learning model means finding the parameters that will fit the training data when running the algorithm to make predictions.
- Problem Framing The first step to developing an ML model is identifying the business case and success criteria. Once these are determined, a plan for achieving the project’s objectives can be created.
- Identify and Extract Data In this step, you need to explore and manage the quality and quantity of data. Therefore, understand how the model will work on real-world data and select and integrate data from several sources. Having good data is vital, as the model will learn from this data.
- Model Naming Select a name for your model. Add a description of the model. Attach appropriate tags to your model. (Tags are designed to make your model searchable.)
- Data Analysis Data analysis is a process that provides a clear understanding of the data and prepares the data to fit the characteristics of the model. Analyzing data helps identify the feature engineering for the ML model. Data preparation is automated for trying out combinations.
- Collect and Prepare the Data In this step, data from several sources is searched and divided (data splits) for training, test sets, and validation. Data has to be modified, assembled, cleaned, and labeled. Also, all duplicates must be removed and all errors corrected. (It’s worth writing functions specifically for this purpose.)
- Select Your Machine Learning Algorithm There are many models from which to choose, depending on the problem. This step includes algorithms of prediction, classification, clustering, deep learning, linear regression, and so on. Experiment with several models from various algorithm categories to find the best-performing model. Perform transformations and feature engineering.
- Train Your Machine Model The goal of training is to answer a question or make a prediction correctly as often as possible. This step involves training the datasets to operate smoothly. For example, algorithms and techniques are involved in training the machine model, such as training with hyperparameters to find the optimal. Also, the model training code is developed during this step.
- Model Quality Evaluation The evaluation step includes selecting the metrics and conducting the actual evaluation. In this step, you evaluate the machine models on the test set, run the pipeline to transform the data, cross-validation, anomaly detection, novelty detection, quality evaluation, etc.
- Model Performance and Adjustment Machine learning models are tested in real-life situations. Data is divided into training and test sets for testing purposes. The model is tested using the “test set” on new instances and checked for generalization errors to determine how the model is overfitting or underfitting the data. At this point, the model should be predicting and ready for deployment.
- Launch, Monitor, and Maintain Now, the model must be deployed to the production environment. ML models need to be monitored with humans or machines to check their performance in order to ensure an optimum data pipeline and data quality. This step involves confirming that the system works with current data and is set to trigger alerts if the model needs retraining.
Scalability of Machine Learning Models
In ML applications, scalability is often a primary concern. Businesses need applications that can maintain the same efficiency when the workload grows, updating to new data and producing predictions. For example, predictions in the stock market happen every millisecond. So, scalability requires building an effective data pipeline. These pipelines should be flexible enough to accommodate many data as well as the high processing velocity required by new ML applications. Therefore, it is essential to make the Machine Learning infrastructure interoperable to incorporate it into the existing and future resources. For this, we need to set up scalable ML applications to increase the systems’ overall performance. Scalable ML algorithms are a class of algorithms that can deal with any amount of data without consuming a tremendous number of resources, such as memory. The primary purpose of scalable algorithms is to allow fast computations for massive data sets.
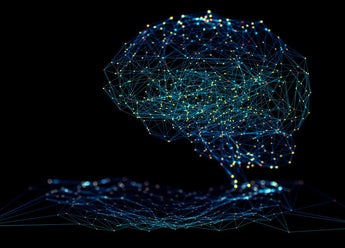
Machine Learning Model Training
The task: Develop an ML training pipeline that describes ML workflows for each ML model and keeps a repository for each model candidate. Completing this task will involve a number of steps:
- Choosing the proper framework and language: ML-based applications can use programming languages such as Python, C++, JavaScript, Java, C#, Julia, Shell, R, TypeScript, and Scala. Python is the most recommended programming language for ML applications. The language can be chosen depending on frameworks, such as TensorFlow, PyTorch, SciKit-Learn, MXNet, Gluon, Sonnet, and Keras. All these frameworks have numerous features. A deep learning framework allows building learning models that are production-ready without getting into the underlying algorithms of the details. Choosing the proper framework that will support your preferred programming language is essential.
- Selecting a suitable processor: Selecting the proper hardware plays a critical role in scalability. In many cases, for ML, the best CPU is a GPU (Graphical Processing Unit), as they are comparatively faster—and quicker in distributing computations across GPU servers. A traditional CPU (Central Processing Unit) is not ideal for large-scale machine learning. Beyond CPU and GPU, there are TPUs (Tensor Processing Units), Google’s custom-developed application-specific integrated circuits, which are used to accelerate machine learning workloads.
- Data collection: Data collection is the process of gathering and measuring data that needs to be formatted, cleansed, reduced, and rescaled to make it better. Data storage is also essential in order to develop solutions for the business problem at hand.
- The Input Pipeline: Data is entered into the learning algorithm as a set of inputs/pipelines. At this stage, the data can be divided (data segregation) into subsets and components, transformed, and then fed it into the system. The data set is then added to the pipeline. Data processing components are self-contained and usually run asynchronously.
- Model Training: A significant step for scalability, model training includes exploring and cleaning the data as well as engineering new features. Training the model means learning good values for all the weights and the bias from labeled examples
- Steps include:
- Inputting training data source
- Naming the data attribute that contains the target to be predicted
- Preparing data transformation instructions
- Training parameters to control the learning algorithm
- Writing a script that runs automatically to train the model
- Testing and validation
- Parameters in Machine Learning:
- Model parameters—set parameters for the model to fit the training set
- Hyperparameters training (write scripts)
- Model Scoring
- Optimization: The final step is to optimize. To achieve that, optimal parameters must be identified. Optimization algorithms check the input parameter to a function that results in the minimum or maximum output. Evaluation of data performance provides estimates on how the model is overfitting or underfitting the training data.
- Machine Learning overfitting happens when the model is too complex and doesn’t perform correctly with the training data, giving generalization errors during the validation set. The ML team should find a balance between bias and variances.
- Machine Learning underfitting is when the model is too simple for the intended dataset and predictions are inaccurate. The model needs additional parameters or, perhaps, more parameters should be added to the features of the algorithm.
- Testing Model: Before the final deployment happens, it is necessary to test whether the input data is in line with the output data and is yielding maximum predictions. These tests—cross-validation, error analysis, and data validation—should be done and monitored multiple times.
- Machine Learning Model Monitoring: The model monitoring phase ensures active performance monitoring to catch errors in production, detect degradation, and ensure consistency of inference data and metrics with business objectives. Monitoring code checks live performance of the models.
- Deploy ML Model: Deployment is when the prediction service goes live. Effectively deploying Machine Learning models is an art rather than a science. Think of deploying frequent model versions of the entire ML system. Cloud hosting platforms are ideal for this purpose. Models can be deployed as a web service and used by web applications that use REST API, deployed as an API for prediction, or containerized. Deploying a model on the cloud using Google AI Cloud Platform provides scalability and load balancing with a perfect environment for running TensorFlow models.
Machine Learning Model Monitoring
Building and managing an integrated ML system that is continuously in production. This process refers to the performance monitoring of ML models to be consumed by business applications. In simple terms, monitoring refers to how the designed and developed models will keep running so that those functions or applications that integrate continue to perform with a high level of accuracy and stability. Operationalization and managing ML models are complex tasks that require maintaining and continuously testing and validating the code, data, and models, managing performance and experiments, and maintaining the accuracy of the algorithms and data to avoid degradation of the models. A model’s success depends on data collection, data engineering, and data science, which means collecting the correct data, understanding the effort needed to extract data from the system, and applying the engineering principles necessary to format, transform, and get the data ready from a data science standpoint. All three lead to the deployment of data.
Machine Learning Pipeline Automation
Machine Learning systems usually create a multistep pipeline that trains and validates models manually. Still, once your team learns and develops a workable model, the ML pipeline matures and must be automated. Automation speeds up new model training and implementations. An ML development and operations culture (MLOps) is needed in order to solve the challenges involved in keeping the ML systems in production continuously. DevOps teams evolve to integrate all the elements with the CI/CD environment in order to automate the building, testing, and deployment of ML pipelines. Continuous training (CT) automation and experimentation in model architecture, feature engineering, and tuning hyperparameters are then added to the operations.
Steps in Automating Machine Learning Model Training
- Evaluation Analyze how to automate the steps of the ML training pipeline to achieve continuous training models from new data for faster iteration and readiness.
- Exploratory Data Analysis (EDA) Data analysis is a manual step to understand the data for building the model before making assumptions. (The model analysis is also a manual task.)
- Build and Test Try algorithms and models and develop the source code for the ML pipeline steps to automate. Build, test, and package components. Source code is sent to the repository. Many types of testing and verifications are performed on the training models.
- Modular Components Components, code, packages, artifacts, and executables are shared for reusability.
- Automated Data Validation Ensures that the expected data behaviors, patterns, and expected features comply with data schema. (Watch trigger alerts.)
- Automated Model Validation With the trained model, test a dataset to verify the quality of the prediction results, checking the values of the variances. Validation is performed offline, and then online validation is handled with canary deployment.
- ML Metadata Metadata is stored when the ML pipeline is executed; the metadata is then used to compare versions during model validation. This process also helps in evaluating metrics, debugging errors, and finding anomalies.
- Triggers Monitoring code is written to check the ML system’s performance and trigger response alerts when detecting changes in data that feed the training model.
- Feature Store A feature store is a repository of features for training and serving that allows the reuse of feature sets with metadata that can be fetched automatically in a batch to the prediction service.
- Verify the Integration and Deploy Verify that configurations are correct for integrating with the target environment (APIs, REST API, etc.) and deploy artifacts. Before deploying, check resources and IT infrastructure.
- Schedule Automatic Execution The trained model is pushed to the registry.
- Prediction Service Model The model is deployed and working with live data.
- Continuous Monitoring Apply continuous testing and verifications to validate and ensure performance.
The process of automating Machine Learning pipelines involves the gradual transition from manual to semi-automated to fully automated. Navigating the timeline for testing and deploying implementations includes a number of different skills and processes.
Dataset in Machine Learning
A dataset is a synchronized collection of data. It can be in the form of a table, a schema, or an object. This process is, once again, an integral part of the Machine Learning process. In simple terms, the word “datasets” means a collection of data. You can usually find a dataset in a tabular form. Each column denotes a specific variable, and each row signifies a specific member of the dataset.
Types of Datasets
There are typically five types of datasets:
- Numerical Dataset A numerical dataset consists of only the numbers—for example, the height and weight of a person, the total number of pages in a notebook, the number of apples in a grocery store, etc.
- Correlation Dataset This type denotes the relationship of variables or attributes between datasets. For example, people that exercise regularly have lower cholesterol levels.
- Multivariate Dataset This dataset consists of multiple variables, such as the length, width, and height of a rectangular box, for example.
- Categorical Dataset This type of dataset consists of the characteristics of a defined object or person, such as an individual’s gender and relationship status, for example.
- Bivariate Dataset This type consists of two variables, such as students’ academic scores and their ages, for example.
Datasets are updated regularly. The most significant benefit of using a dataset is that it helps the user obtain desired data in an organized manner, retrieving the required information quickly from a massive collection of data, thereby saving time and executing tasks more quickly.
Machine Learning Models in Python
Python is particularly suitable for building Machine Learning models and applications. Intuitive and easy to read, it is supported by an extensive collection of ML libraries and frameworks. Get started and learn how to train a machine learning model in Python.
- Get Started Learning Python
- The Python Tutorial
- Python Libraries (NumPy, Pandas, Matplotlib, etc.)
Python Frameworks
Production-ready Python frameworks include:
Challenges with Machine Learning Systems
- Requires continuous testing and validation of data and models
- Needs to integrate external solutions to the ML pipeline
- Model analysis and retraining of candidate ML model
- MLOps culture implementation throughout the machine learning development lifecycle
- Process and metadata management
- Automate the steps of building the ML model
- Data collection and verification
- Retraining models due to degradation or decay (tracking statistics and looking for emerging patterns)
- Tracking experiments to reproduce and reuse during the lifecycle
Machine Learning Model Development
You can build and deploy ML models and manage your Machine Learning operations (MLOps) with a collaborative partnership with Krasamo. Our expert teams can implement your ML operations through third-party services such as AWS or Google. This modality simplifies and helps manage your workflows, thereby avoiding infrastructure management tasks.
- Build ML pipeline using TensorFlow
- Evaluate and monitor model performance
- Metadata management (metadata tracking)
- Track artifacts and lineage
- Collaboration capabilities
- Feature engineering
- Track data and performance (notifications)
Machine learning has become an integral part of business operations in the digital age, together with large amounts of data and computing power. Have you been wondering how to power your products and features through Machine Learning? Or how to transform your business in disruptive environments? Machine Learning is particularly suitable for products that require solving complex problems and typically demand high human involvement for fine-tuning and analyzing large amounts of data. Machine Learning helps product managers improve products and product offerings by mining data and using ML models to find new patterns from predictions that were previously unknown or difficult to see. A successful Machine Learning strategy emphasizes business issues to solve, builds a business case, and puts users at the center while applying the relevant technical aspects to the project.